What Is GPT and How Will It Be Used for Customer Service?
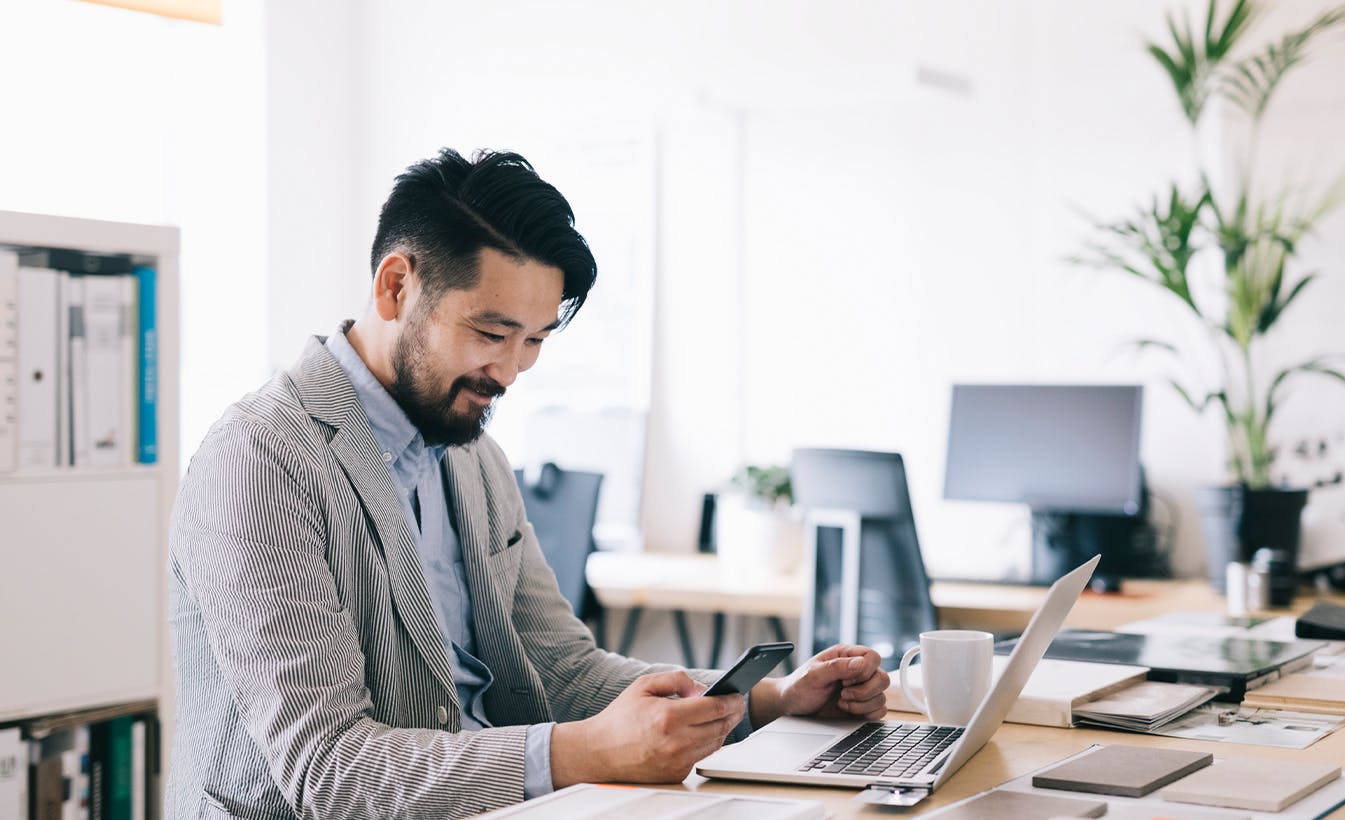
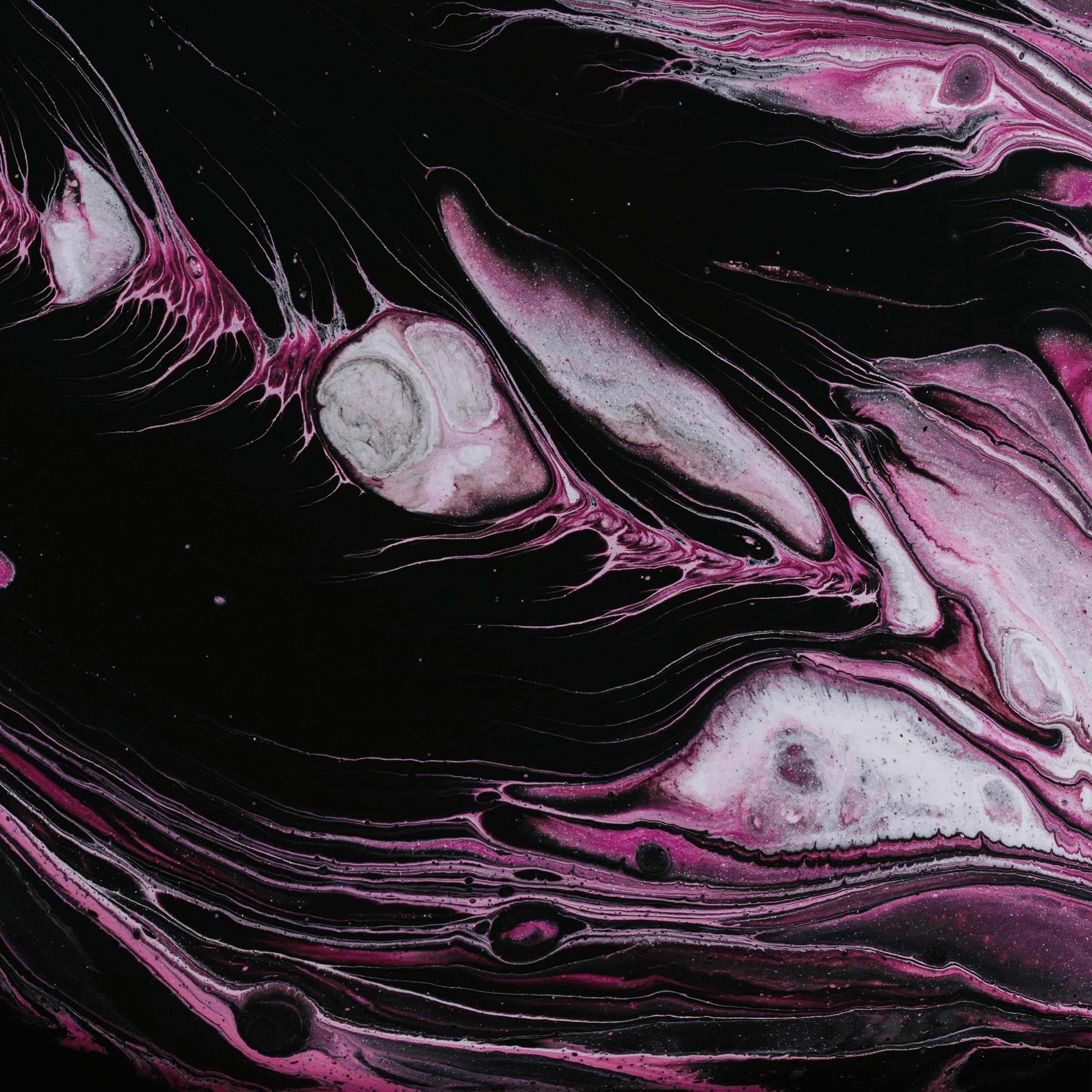
This post was co-authored by Ian Beaver and Scott Lindsay.
You’ve likely heard about GPT (generative pre-trained transformer) based artificial intelligence. You may have seen OpenAI’s family of these models—ChatGPT—in the news lately and wondered, “What is GPT?”.
Most customer experience (CX) executives and specialists understand how these platforms (GPT-3, Gemini, Claude, etc.) can emulate human conversation, the writing of long-deceased historical figures, or help teachers prevent students from plagiarizing research papers. There’s even talk that GPT-based programs could someday detect Alzheimer’s.
This is an exciting evolution of conversational AI, to be sure. But when it comes to conversational AI for business, companies are left wondering how we’ll use GPT for customer support and other corporate roles.
More specifically, how will it work with call centers, self-service, and other customer engagement needs?
Read on to find out.
What is GPT?
GPT stands for Generative Pre-trained Transformer. It is a type of neural network architecture developed by OpenAI that is trained on large datasets unsupervised and can generate human-like text.
Some key things to know about GPT models:
- GPT models are auto-regressive. These platforms generate text one word at a time based on the previous words. This allows them to model context and produce fluent and coherent text.
- They are pre-trained on large, unlabeled text datasets. Each model uses a self-supervised objective called masked language modeling to acquire broad linguistic knowledge from the data.
- GPT models are complex. Often, these systems can contain close to a hundred billion parameters. The larger size of these models makes them highly efficient in generating more coherent long-form text and conversational AI.
- GPT models expand AI capabilities. Tools like chatbots, creative writing assistants, and code generators continue to showcase artificial intelligence’s natural language processing capabilities.
Learning these details about what GPT is and how it operates makes it easy to see how this all plays out in the real world. Imagine the benefits this could provide as we apply use cases of GPT for customer service.
How GPT Works
GPT models construct conversational AI answers to users’ queries using context and sentence sequencing knowledge gained during the pre-training phase. Below is a more detailed explanation of how that occurs.
Architecture
At its core, GPT models utilize a decoder-only transformer architecture to generate text word-by-word. The transformer leverages multi-headed self-attention mechanisms to model the contextual relationships between all words, allowing it to focus on relevant previous context.
Multiple transformer decoder blocks are stacked, providing model depth to capture intricate language representations. Combined with broad unsupervised pretraining, this decoder architecture gives GPT the foundation to produce fluent, coherent text tailored to the initial prompt by predicting subsequent tokens autoregressively. The scale of parameters in the hundreds of billions further enables human-like generation capabilities.
Training
GPT models are trained on vast text datasets through a self-supervised process called masked language modeling. Random words from the input text are masked, and GPT learns to predict the original masked words based on the surrounding context. This allows GPT to deeply understand the patterns and structure of language from the dataset.
The model parameters are updated through backpropagation to improve accuracy on this masking task, creating an implicit understanding of language. This pre-training is key to enabling GPT’s fluent text generation capabilities.
Generating Text
GPT models generate text recursively and autoregressively using the initial user-supplied prompt as context. Specifically, they leverage this seed prompt to predict the next word based on probability. This word is added to the output, updating context.
GPT repeats this process – predicting the next word based on context, appending the chosen word to the sequence, and sliding the context forward – generating a stream of text blossoming from the initial prompt. As words accumulate, concepts emerge in the text output itself. The platform also incorporates these concepts into its context to improve coherence.
Enhancing context in this way allows multi-paragraph passages to flow logically from the few sentences supplied to the model. Ultimately, the depth of the pre-training data will determine the flexibility of text generation – i.e., the length possible while maintaining language fluency and topic relevance according to the prompt.
Our guide to conversational AI takes a deeper look at this process for those interested in learning more about GPT’s cutting-edge technology.
ChatGPT and Your Business
At Verint, we know that the race toward fully realized conversational AI can’t just be about speaking the language and understanding all things in general terms. Instead, the conversational AI model needs to know and understand how businesses operate, the terms they use, and the context in which they use them.
A truly intelligent GPT model deciphers how those terms drive decisions through an organization’s ecosystems of business applications.
And while ChatGPT is advanced and promising in so many ways, it’s not a plug-and-play solution for customer service quite yet.
Imagine ChatGPT as a new employee in your contact center. If you ask that employee to summarize call interactions on their first day, they’ll be able to generate sentences and explanations that are generally accurate but may seem suspect or incomplete to the experts on your team.
Asking that same individual to do the job two months later – when they’re starting to understand how the business operates and what customers want – and their summaries will be more actionable, categorical, and specific to the terms that the client uses to run its business.
The problem with conversational AI chatbots like ChatGPT is they can’t immediately jump the learning curve necessary to deliver accurate solutions to complex business challenges.
While its responses may sound impressive, it has not been trained on your business and cannot answer questions about your products or services—such as if a warranty claim can be accepted or if a customer’s insurance policy will cover their latest claim.
Worse, GPT models may provide factual inaccuracies to negative customer service interactions like scheduling an appointment at the wrong medical clinic, or depositing money to the wrong account.
Neither your business’ reputation nor its bottom line can survive sending out bad information—and know that customers won’t be forgiving just because that bad information came from a bot rather than a live agent.
In today’s world, it’s one and the same.
Verint and GPT Models
As a leader in conversational AI technology, Verint is very much aware of how it can help your business. We’ve evaluated the results of several generative language model technologies for multiple use cases within our realm of expertise. This research is the data behind Verint Da Vinci AI—the technology that powers many of our automated customer engagement solutions.
Da Vinci is built to swiftly incorporate any evolving or revolutionary commercially available technology into the Verint Customer Engagement Platform. However, it’s important to note that GPT models can’t evolve without experts like we have at Verint injecting proficiency and specific knowledge into them. We understand the business ecosystems surrounding the contact center and how decisions are made and driven forward through those systems.
Verint Da Vinci focuses on creating a business translation layer so your bots can speak in terms specific to your business and brand. Here are a few of the tools that make that happen:
- Intent Classifier: Predict the speaker’s intention for an utterance, incorporating specific knowledge of your business terminology
- Interaction Analytics: Identify the purpose, disposition, resolution, and actions related to a specific interaction
- Automated Quality Management: Use AI to automate a quality management audit of an interaction and provide a comparative score for agent improvements
- Outcome Prediction: Use call data to predict KPIs such as satisfaction (CSAT), likelihood-to-recommend (LTR) and Net Promoter Score (NPS), churn, or customer effort scores.
Embracing AI Evolution
Verint embraces the evolution of conversational AI technology—and we’re excited to be part of that evolution ourselves.
Our expertise in what GPT is, as well as other early emergers like neural symbolic models, has enormous potential in helping us provide applications that address what we call Engagement Capacity Gap—the continuing divide between customer expectations and the resources your company has to meet those expectations.
Want to learn more about how Verint Da Vinci AI and Verint Conversational AI are driving excellent customer engagement around the globe? We’d be happy to show you more.